
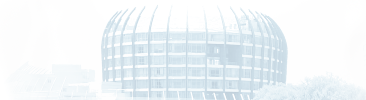
主講人🛹:沈建成
地點♥︎:沐鸣娱乐松江校區1號沐鸣樓240室
組織單位:沐鸣注册
報告人簡介:
沈建成🍡,蘇州大學商沐鸣金融系特聘教授,博士生導師,瑞士蘇黎世大學訪問教授。曾分別在美國Regent University, Taylor University擔任金融教職。研究領域為實證行為金融👩🔬♚,理論資產定價,人工智能大數據,神經實驗經濟學💆🏽♂️🧈。近年來發表了 20多篇國際高水平期刊論 文, 諸 如 Computer Networks, Journal of Banking and Finance,Journal of Financial Research, Journal of Futures Market, Journal of Real Estate Finance and Economics, Journal of Behavioral Finance, Information and Management 等🧪。此外,擔任了 Journal of Asset Management, Journal of Business Research, Scientific Reports, 瑞士國家自然基金的審稿人。
報告摘要👰🏽:
Investor psychology provides an important avenue for modeling non-fundamental behaviors in financial analysis. Yet, whether market psychological information has a practical application in predicting asset returns is still under debate. Thus, a burgeoning number of machine learning algorithms have been developed to test the effectiveness of investor psychology in financial predictions. With all the merits of machine learning approach, the drawbacks are prediction biases, data overfitting issues and poor performance. To address these issues, we developed a DeepPsych system to harness the power of high frequency TRMI psychology data for market prediction. In a “hybridization–generalization–dual-channel-fusion” three-stage experiment, we evaluate each proposed module and the entire framework against the state-of-art machine learning benchmarks on investor psychology and trading data of the SPY (SP500 ETF). Results demonstrate that our deep learning framework can automatically identify features that are more effective than fundamental factors and support profitable trading.
投資者心理學為金融分析中的非基本行為建模提供了一條重要途徑🖇。然而,市場心理信息在預測資產回報方面是否具有實際應用仍存在爭議。因此🟩,已經開發了大量機器學習算法來測試投資者心理在財務預測中的有效性。盡管機器學習方法具有所有優點🧑🏻🔧,但缺點是預測偏差、數據過度擬合問題和性能不佳。為了解決這些問題,我們開發了一個 DeepPsych 系統🪈,利用高頻 TRMI 心理數據的力量進行市場預測🧊。在“混合-泛化-雙通道融合”三階段實驗中,我們根據最先進的機器學習基準評估了每個提出的模塊和整個框架👐🏻,這些基準涉及投資者心理和SPY(SP500 ETF)的交易數據👎。結果表明,我們的深度學習框架可以自動識別特征數據並達成盈利的交易。
關鍵詞👨🏻💻:投資者心理🏌️,深度學習𓀘,高頻交易系統
主持人:陶然